Mathematical statistics is a methodology that allows you to make informed decisions in the face of uncertain conditions. The study of methods for collecting and systematizing data, processing the final results of experiments and experiments with mass randomness, and discovering any patterns is what this branch of mathematics does. Consider the basic concepts of mathematical statistics.
Difference with probability theory
Methods of mathematical statistics closely intersect with probability theory. Both branches of mathematics deal with the study of numerous random phenomena. The two disciplines are connected by limit theorems. However, there is a big difference between these sciences. If the probability theory determines the characteristics of a process in the real world on the basis of a mathematical model, then mathematical statistics does the opposite - it sets the properties of the model tobased on observed information.
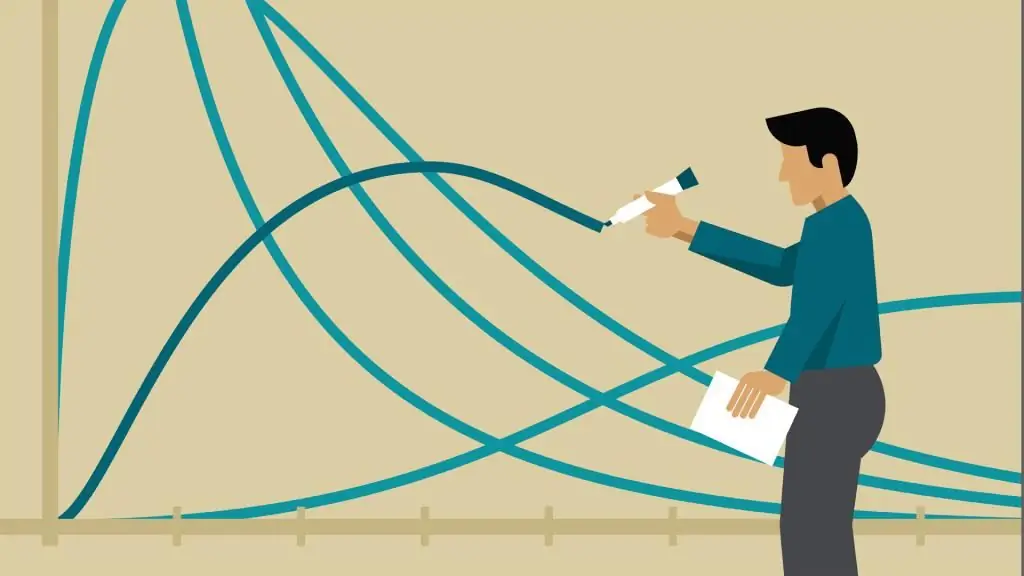
Steps
The application of mathematical statistics can be carried out only in relation to random events or processes, or rather, to data obtained from observing them. And this happens in several stages. First, the data of experiments and experiments undergo certain processing. They are ordered for clarity and ease of analysis. Then an exact or approximate estimate of the required parameters of the observed random process is made. They can be:
- assessment of the probability of an event (its probability is initially unknown);
- studying the behavior of an indefinite distribution function;
- expectation estimate;
- variance estimation
- etc.
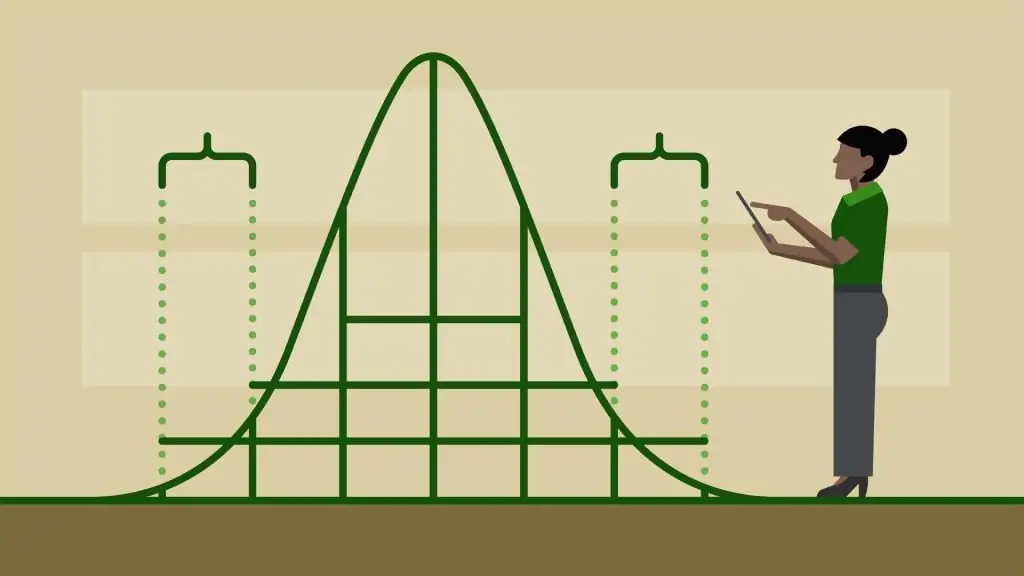
The third stage is the verification of any hypotheses set before the analysis, i.e., obtaining an answer to the question of how the results of the experiments correspond to the theoretical calculations. In fact, this is the main stage of mathematical statistics. An example would be to consider whether the behavior of an observed random process is within the normal distribution.
Population
The basic concepts of mathematical statistics include general and sample populations. This discipline deals with the study of a set of certain objects regarding some property. An example is the work of a taxi driver. Consider these random variables:
- load or number of customers: per day, before lunch, after lunch, …;
- average travel time;
- number of incoming applications or their attachment to city districts and much more.
It is also worth noting that it is possible to study a set of similar random processes, which will also be a random variable that can be observed.
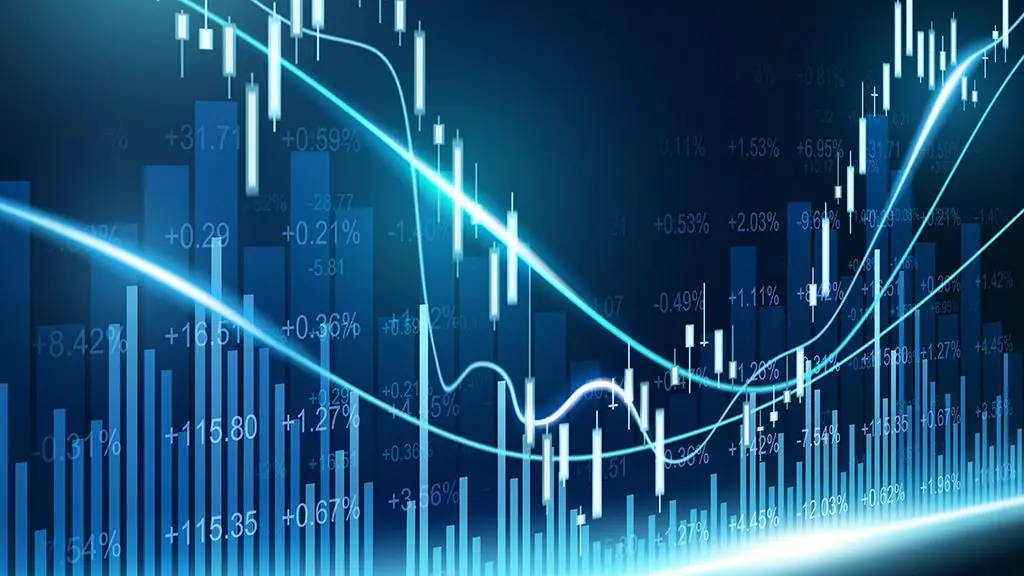
So, in the methods of mathematical statistics, the whole set of objects under study or the results of various observations that are carried out under the same conditions on a given object is called the general population. In other words, mathematically more strictly, it is a random variable that is defined in the space of elementary events, with a class of subsets designated in it, the elements of which have a known probability.
Sample population
There are cases when it is impossible or impractical for some reason (cost, time) to conduct a continuous study to study each object. For example, opening every jar of sealed jam to check its quality is a dubious decision, and trying to estimate the trajectory of each air molecule in a cubic meter is impossible. In such cases, the method of selective observation is used: a certain number of objects are selected (usually randomly) from the general population, and they are subjected to their analysis.
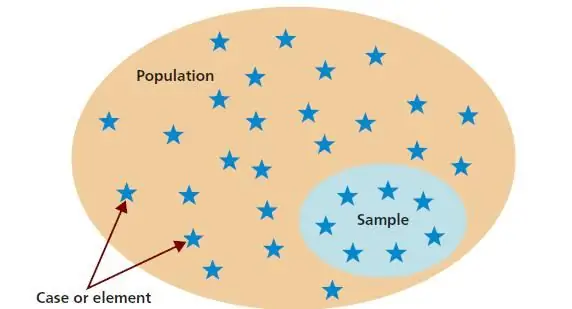
These concepts may seem complicated at first. Therefore, in order to fully understand the topic, you need to study the textbook by V. E. Gmurman "Probability Theory and Mathematical Statistics". Thus, a sampling set or sample is a series of objects selected at random from the general set. In strict mathematical terms, this is a sequence of independent, uniformly distributed random variables, for each of which the distribution coincides with that indicated for the general random variable.
Basic concepts
Let's briefly consider a number of other basic concepts of mathematical statistics. The number of objects in the general population or sample is called volume. The sample values that are obtained during the experiment are called the sample realization. In order for an estimate of the general population based on a sample to be reliable, it is important to have a so-called representative or representative sample. This means that the sample must fully represent the population. This can only be achieved if all elements of the population have an equal probability of being in the sample.
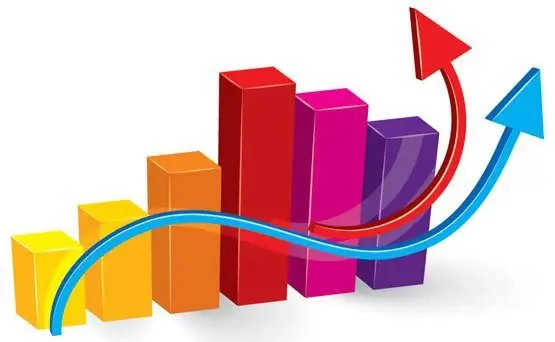
Samples distinguish between return and non-return. In the first case, in the content of the sample, the repeated element is returned to the general set, in the second, it is not. Usually, in practice, sampling without replacements is used. It should also be noted that the size of the general population always significantly exceeds the size of the sample. Existmany options for the sampling process:
- simple - items are randomly selected one at a time;
- typed - the general population is divided into types, and a choice is made from each; an example is a survey of residents: men and women separately;
- mechanical - for example, select every 10th element;
- serial - selection is made in series of elements.
Statistical distribution
According to Gmurman, probability theory and mathematical statistics are extremely important disciplines in the scientific world, especially in its practical part. Consider the statistical distribution of the sample.
Suppose we have a group of students who were tested in mathematics. As a result, we have a set of estimates: 5, 3, 1, 4, 3, 4, 2, 5, 4, 4, 5 - this is our primary statistical material.
First of all, we need to sort it, or perform a ranking operation: 1, 2, 3, 3, 4, 4, 4, 4, 5, 5, 5 - and thus get a variational series. The number of repetitions of each of the assessments is called the assessment frequency, and their ratio to the sample size is called the relative frequency. Let's make a table of the statistical distribution of the sample, or just a statistical series:
ai | 1 | 2 | 3 | 4 | 5 |
pi | 1 | 1 | 2 | 4 | 3 |
or
ai | 1 | 2 | 3 | 4 | 5 |
pi | 1/11 | 1/11 | 2/11 | 4/11 | 3/11 |
Let's have a random variable on which we will conduct a series of experiments and see what value this variable takes. Suppose she took the value a1 - m1 times; a2 - m2 times, etc. The size of this sample will be m1 + … + mk=m. The set ai, where i varies from 1 to k, is a statistical series.
Interval distribution
In the book by VE Gmurman "Probability Theory and Mathematical Statistics" an interval statistical series is also presented. Its compilation is possible when the value of the feature under study is continuous in a certain interval, and the number of values is large. Consider a group of students, or rather, their height: 163, 180, 185, 172, 161, 171, 189, 157, 165, 174, 180, 181, 175, 182, 167, 159, 173, 171, 164, 179, 160, 180, 166, 178, 156, 180, 189, 173, 174, 175 - 30 students in total. Obviously, the height of a person is a continuous value. We need to define the interval step. For this, the Sturges formula is used.
h= | max - min | = | 190 - 156 | = | 33 | = | 5, 59 |
1+log2m | 1+log230 | 5, 9 |
Thus, the value of 6 can be taken as the size of the interval. It should also be said that the value 1+log2m is the formula fordetermining the number of intervals (of course, with rounding). Thus, according to the formulas, 6 intervals are obtained, each of which has a size of 6. And the first value of the initial interval will be the number determined by the formula: min - h / 2=156 - 6/2=153. Let's make a table that will contain intervals and the number of students whose growth fell within a certain interval.
H | [153; 159) | [159; 165) | [165; 171) | [171; 177) | [177; 183) | [183; 189) |
P | 2 | 5 | 3 | 9 | 8 | 3 |
P | 0, 06 | 0, 17 | 0, 1 | 0, 3 | 0, 27 | 0, 1 |
Of course, this is not all, because there are much more formulas in mathematical statistics. We have considered only some basic concepts.
Distribution schedule
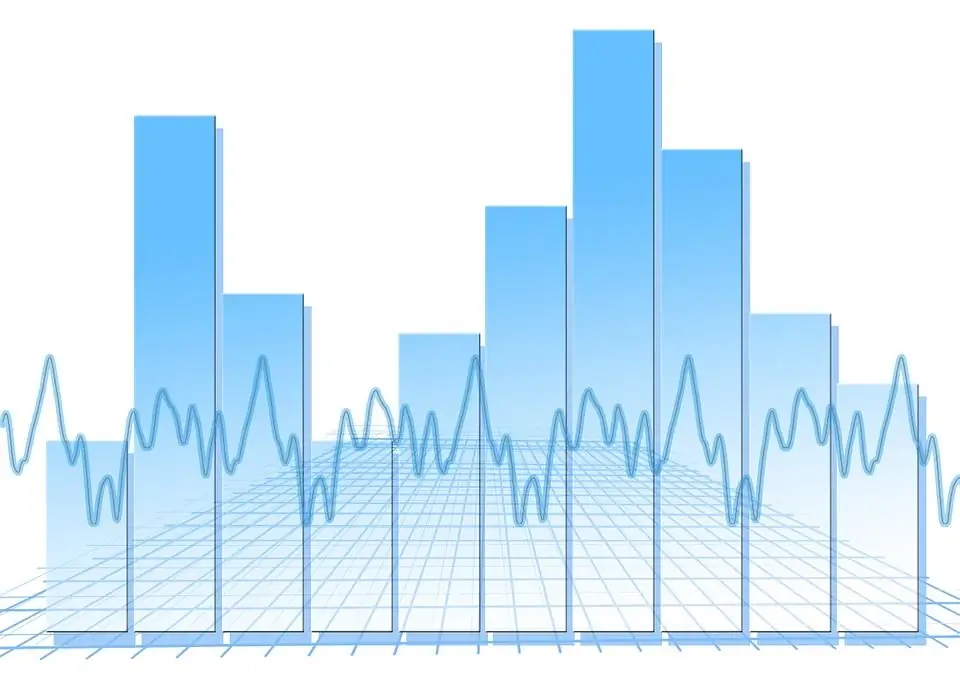
The basic concepts of mathematical statistics also include a graphical representation of the distribution, which is distinguished by clarity. There are two types of graphs: polygon and histogram. The first is used for a discrete statistical series. And for continuous distribution, respectively, the second one.