Hypothesis testing is a necessary procedure in statistics. A hypothesis test evaluates two mutually exclusive statements to determine which statement is best supported by the sample data. When a finding is said to be statistically significant, it is due to a hypothesis test.
Verification methods
Methods for testing statistical hypotheses are methods of statistical analysis. Typically, two sets of statistics are compared, or a sampled data set is compared with a synthetic data set from an idealized model. The data must be interpreted in such a way as to add new meanings. You can interpret them by assuming a certain structure of the final result and using statistical methods to confirm or reject the assumption. The assumption is called a hypothesis, and the statistical tests used for this purpose are called statistical hypotheses.
H0 and H1 hypotheses
There are two mainthe concepts of statistical testing of hypotheses - the so-called "main, or null hypothesis" and " alternative hypothesis". They are also called Neyman-Pearson hypotheses. The statistical test assumption is called the null hypothesis, the main hypothesis, or H0 for short. It is often referred to as the default assumption or the assumption that nothing has changed. A test assumption violation is often referred to as the first hypothesis, alternative hypothesis, or H1. H1 is shorthand for some other hypothesis, because all we know about it is that the H0 data can be discarded.
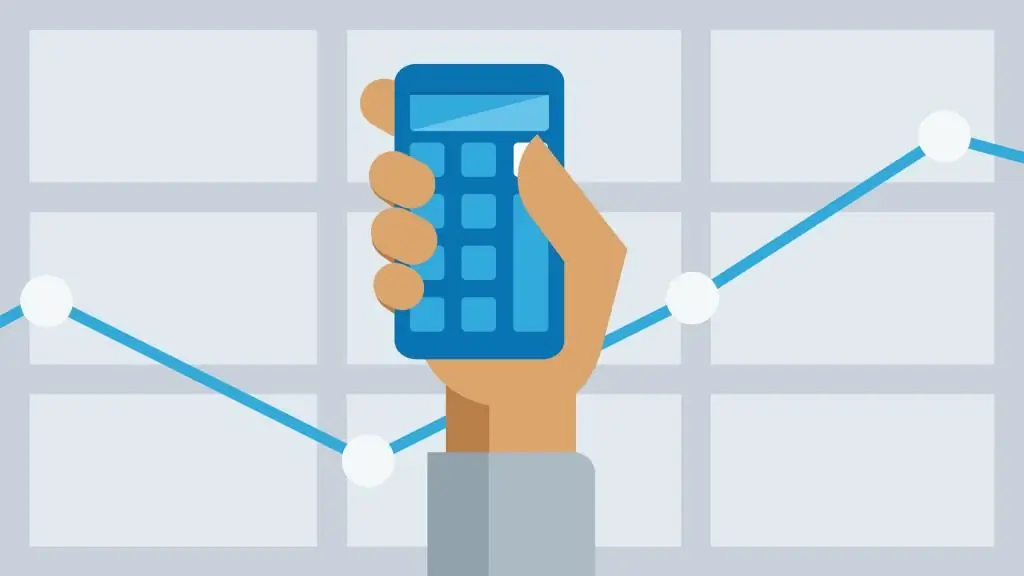
Before rejecting or not rejecting the null hypothesis, the test result must be interpreted. A comparison is considered statistically significant if the relationship between the datasets is unlikely to be the implementation of the null hypothesis according to the threshold probability - the level of significance. There are also goodness-of-fit criteria for statistical hypothesis testing. This is the name of the hypothesis test criterion, which is associated with the supposed law of the unknown distribution. This is a numerical measure of the discrepancy between the empirical and theoretical distributions.
Procedure and criteria for testing statistical hypotheses
The most common hypothesis selection methods are based on either the Akaike information criterion or the Bayesian coefficient. Statistical hypothesis testing is a key technique in both inference and Bayesian inference, although the two types have notable differences. Statistical Hypothesis Testsdefine a procedure that controls the probability of erroneously deciding on an incorrect default or null hypothesis. The procedure is based on how likely it is to work. This probability of making a wrong decision is the improbability that the null hypothesis is true and that no particular alternative hypothesis exists. The test cannot show whether it is true or false.
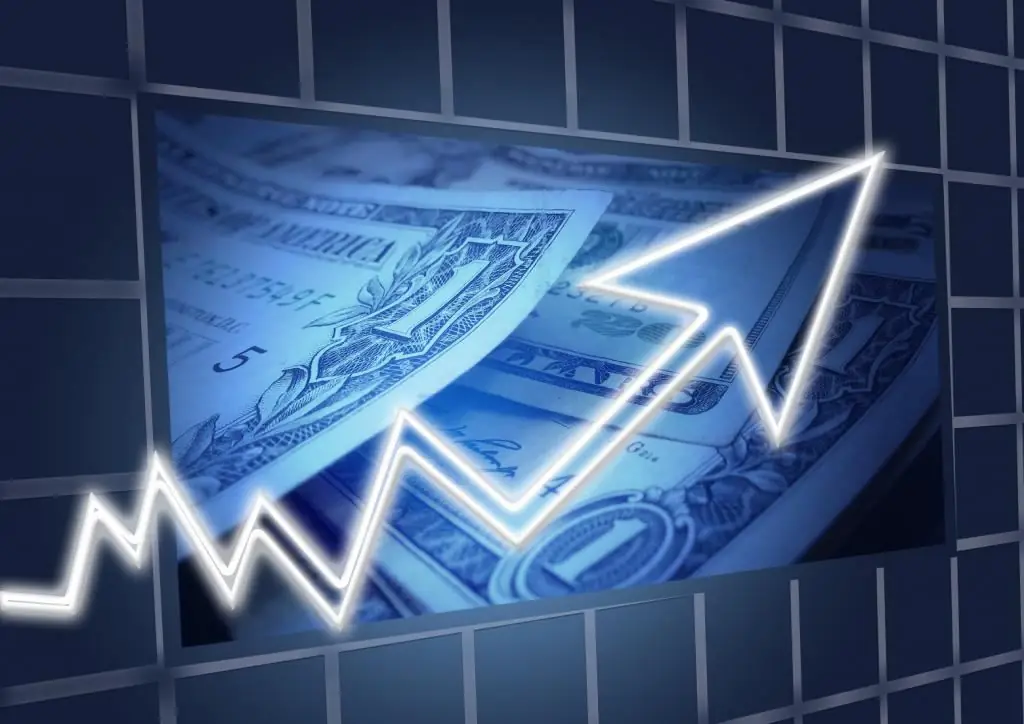
Alternative methods of decision theory
Alternative methods of decision theory exist, in which the null and first hypotheses are considered on a more equal footing. Other decision-making approaches, such as Bayesian theory, attempt to balance the consequences of bad decisions across all possibilities rather than focusing on a single null hypothesis. A number of other approaches to deciding which of the hypotheses is correct rely on the data, which of them have desirable properties. But hypothesis testing is the dominant approach to data analysis in many fields of science.
Testing the statistical hypothesis
Whenever one set of results differs from another set, one must rely on statistical hypothesis testing or statistical hypothesis tests. Their interpretation requires a proper understanding of p-values and critical values. It is also important to understand that, regardless of the level of significance, tests may still contain errors. Therefore, the conclusion may not be correct.
The testing process consists ofmultiple steps:
- An initial hypothesis is being created for research.
- Relevant null and alternative hypotheses are indicated.
- Explains statistical assumptions about the sample in the test.
- Determining which test is appropriate.
- Select the significance level and the probability threshold below which the null hypothesis will be rejected.
- The distribution of the null hypothesis test statistic shows the possible values at which the null hypothesis is rejected.
- Calculation in progress.
- A decision is made to reject or accept the null hypothesis in favor of an alternative.
There is an alternative that uses a p-value.
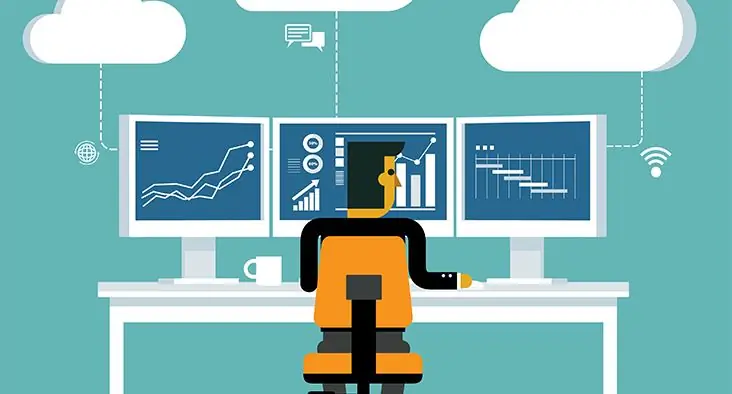
Significance tests
Pure data is of no practical use without interpretation. In statistics, when it comes to asking questions about data and interpreting results, statistical methods are used to ensure the accuracy or likelihood of answers. When testing statistical hypotheses, this class of methods is called statistical testing, or significance tests. The term “hypothesis” is reminiscent of scientific methods, where hypotheses and theories are investigated. In statistics, a hypothesis test results in a quantity given a given assumption. It allows you to interpret whether an assumption is true or a violation has been made.
Statistical interpretation of tests
Hypothesis testsare used to determine which research results will lead to the rejection of the null hypothesis for a predetermined level of significance. The results of a statistical hypothesis test must be interpreted so that work can continue on it. There are two common forms of statistical hypothesis testing criteria. These are p-value and critical values. Depending on the selected criterion, the results obtained must be interpreted differently.
What is a p-value
Output is described as statistically significant when interpreting the p-value. In fact, this indicator means the probability of error if the null hypothesis is rejected. In other words, it can be used to name a value that can be used to interpret or quantify a test result, and to determine the probability of error in rejecting the null hypothesis. For example, you can run a normality test on a sample of data and find that it is unlikely to be outlier. However, the null hypothesis does not have to be abandoned. A statistical hypothesis test may return a p-value. This is done by comparing the value of p against a predetermined threshold value called the significance level.
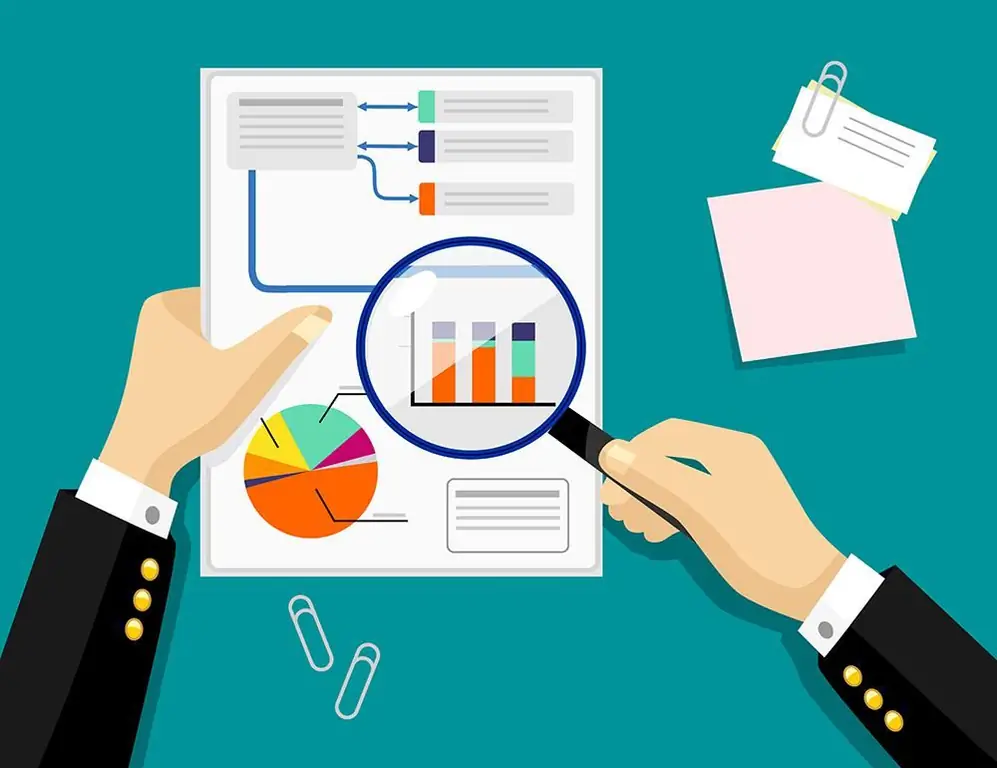
Level of Significance
The level of significance is often written with the Greek lowercase letter "alpha". The general value used for alpha is 5%, or 0.05. A smaller alpha value suggests a more robust interpretation of the null hypothesis. The p-value is compared topreselected alpha value. The result is statistically significant if the p-value is less than alpha. The significance level can be inverted by subtracting it from one. This is done to determine the confidence level of the hypothesis given the observed sample data. When using this method of testing statistical hypotheses, the P-value is probabilistic. This means that in the process of interpreting the result of a statistical test, one does not know what is true or false.
Statistical hypothesis testing theory
Rejection of the null hypothesis means that there is enough statistical evidence that it looks likely. Otherwise, it means that there are not enough statistics to reject it. One can think of statistical tests in terms of the dichotomy of rejecting and accepting the null hypothesis. The danger of a statistical test for testing the null hypothesis is that, if accepted, it may appear to be true. Instead, it would be more correct to say that the null hypothesis is not rejected because there is not enough statistical evidence to reject it.
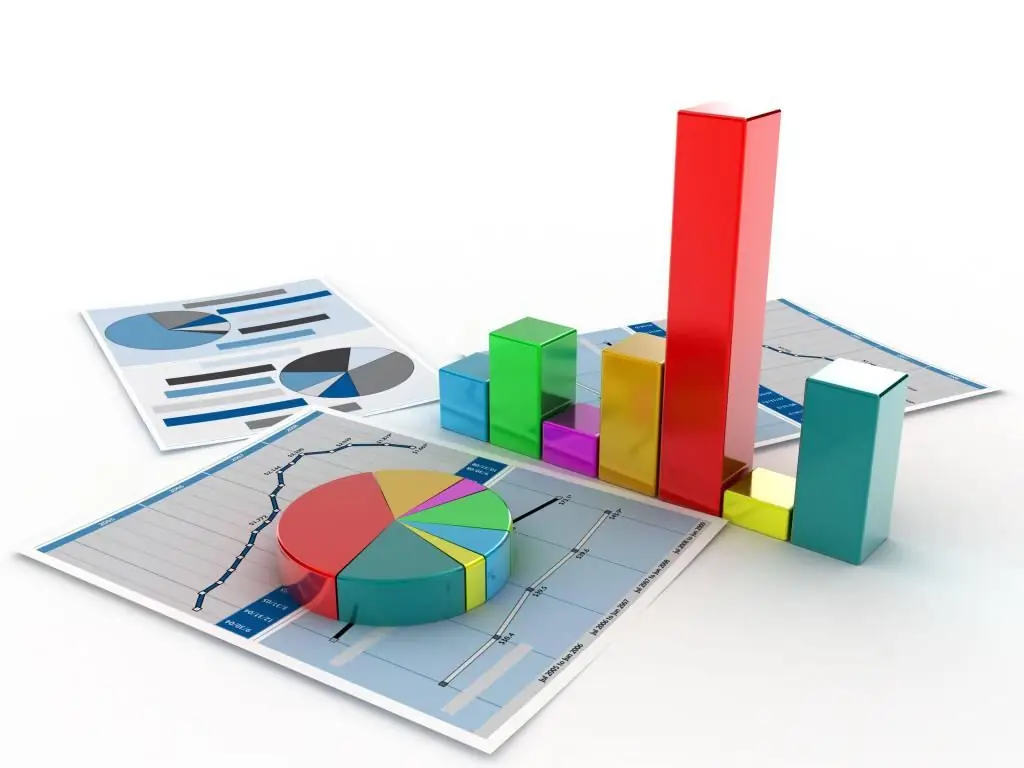
This moment often confuses novice extras. In such a case, it is important to remind yourself that the result is probabilistic and that even accepting the null hypothesis still has a small chance of error.
True or false null hypothesis
Interpretation of the value of p does not mean that zerothe hypothesis is true or false. This means that a choice has been made to reject or not reject the null hypothesis at a certain level of statistical significance based on the empirical data and the chosen statistical test. Therefore, the p-value can be thought of as the probability of the data given under a predetermined assumption embedded in the statistical tests. The p-value is a measure of how likely the data sample will be observed if the null hypothesis is true.
Interpretation of critical values
Some tests don't return p. Instead, they may return a list of critical values. The results of such a study are interpreted in a similar way. Instead of comparing a single p-value with a predetermined level of significance, the test statistic is compared to a critical value. If it turns out to be less, it means that it was not possible to reject the null hypothesis. If greater than or equal, the null hypothesis should be rejected. The meaning of the statistical hypothesis testing algorithm and the interpretation of its result is similar to the p-value. The level of significance chosen is a probabilistic decision to reject or not reject the base test assumption given the data.
Errors in statistical tests
The interpretation of a statistical hypothesis test is probabilistic. The task of testing statistical hypotheses is not to find a true or false statement. Test evidence may be erroneous. For example, if the alpha was 5%, this means that for the most part 1 out of 20the null hypothesis will be rejected by mistake. Or it won't because of the statistical noise in the data sample. Given this point, a small p value at which to reject the null hypothesis may mean that it is false or that an error has been made. If this type of error is made, the result is called a false positive. And such an error is an error of the first kind when testing statistical hypotheses. On the other hand, if the p-value is large enough to mean rejection of the null hypothesis, it may mean that it is true. Or is not correct, and some unlikely event occurred due to which the error was made. This type of error is called a false negative.
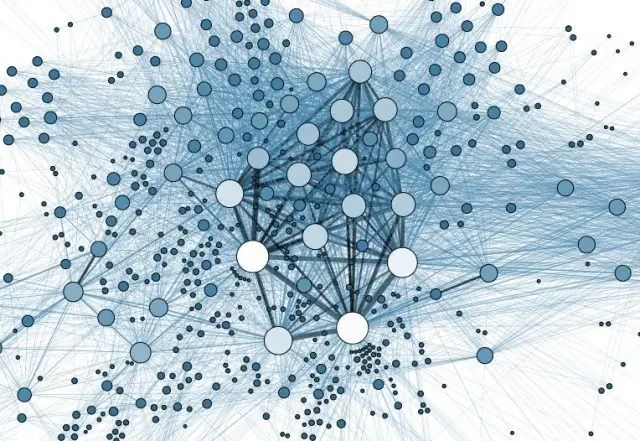
Probability of errors
When testing statistical hypotheses, there is still a chance of making any of these types of errors. False data or false conclusions are quite likely. Ideally, a significance level should be chosen that minimizes the likelihood of one of these errors. For example, statistical testing of null hypotheses may have a very low level of significance. Although significance levels such as 0.05 and 0.01 are common in many fields of science, the most commonly used significance level is 310^-7, or 0.0000003. It is often referred to as “5-sigma”. This means that the conclusion was random with a probability of 1 in 3.5 million independent repetitions of the experiments. Examples of testing statistical hypotheses often carry such errors. This is also the reason why it is important to have independent results.verification.
Examples of using statistical verification
There are several common examples of hypothesis testing in practice. One of the most popular is known as “Tea Tasting”. Dr. Muriel Bristol, a colleague of biometrics founder Robert Fisher, claimed to be able to tell for sure whether it was added first to a cup of tea or milk. Fisher offered to give her eight cups (four of each variety) at random. The test statistic was simple: counting the number of successes in choosing a cup. The critical region was the only success out of 4, possibly based on the usual probability criterion (< 5%; 1 in 70 ≈ 1.4%). Fisher argued that an alternative hypothesis is not required. The lady correctly identified each cup, which was considered a statistically significant result. Fisher's book "Statistical Methods for Researchers" was born from this experience.
Defendant example
The statistical trial procedure is comparable to a criminal court where the defendant is presumed innocent until proven guilty. The prosecutor tries to prove the guilt of the defendant. Only when there is sufficient evidence for a charge can the defendant be found guilty. At the beginning of the procedure, there are two hypotheses: "The defendant is not guilty" and "The defendant is guilty." The hypothesis of innocence can only be rejected when error is very unlikely because one does not want to convict an innocent defendant. Such an error is called a Type I error, and its occurrencerarely controlled. As a consequence of this asymmetric behavior, Type II error, i.e. acquittal of the perpetrator, is more common.
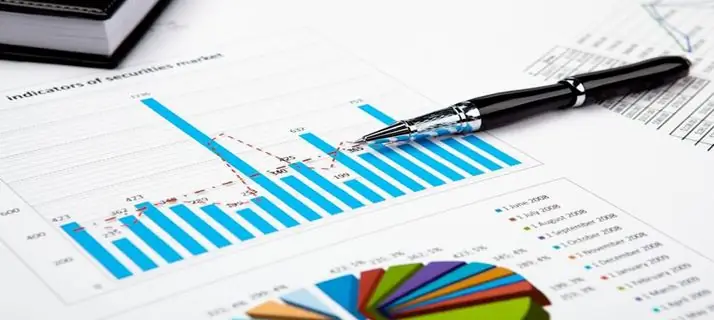
Statistics are useful when analyzing large amounts of data. This applies equally to the testing of hypotheses, which can justify the conclusions even if no scientific theory exists. In the tea tasting example, it was "obvious" that there was no difference between pouring milk into tea or pouring tea into milk.
Real practical application of hypothesis testing includes:
- testing whether men have more nightmares than women;
- document attribution;
- Assessing the influence of the full moon on behavior;
- determining the range in which a bat can detect an insect using an echo;
- choosing the best means to quit smoking;
- checking if bumper stickers reflect the behavior of the car owner.
Statistical hypothesis testing plays an important role in statistics in general and in statistical inference. Value testing is used as a replacement for the traditional comparison of predicted value and experimental result at the core of the scientific method. When a theory is only capable of predicting the sign of a relationship, directed hypothesis tests can be configured in such a way that only a statistically significant result supports the theory. This form of evaluation theory is the most rigidcriticism of the use of hypothesis testing.