Throughout the history of statistics, various attempts have been made to create a taxonomy of levels of measurement. Psychophysicist Stanley Smith Stevens defined nominal, ordinal, interval, and proportional scales.
Nominal measurements have no significant order of ranks among values and allow any one-to-one conversion.
Regular dimensions have inexact differences between consecutive values, but have a specific order of those values and allow any order-preserving transformation.
Interval measurements have meaningful distances between points, but the zero value is arbitrary (as in the case of longitude and temperature measurements in Celsius or Fahrenheit) and allows for any linear transformation.
Ratio dimensions have both a meaningful zero value and distances between different dimensions, and allow for any scaling transformation.
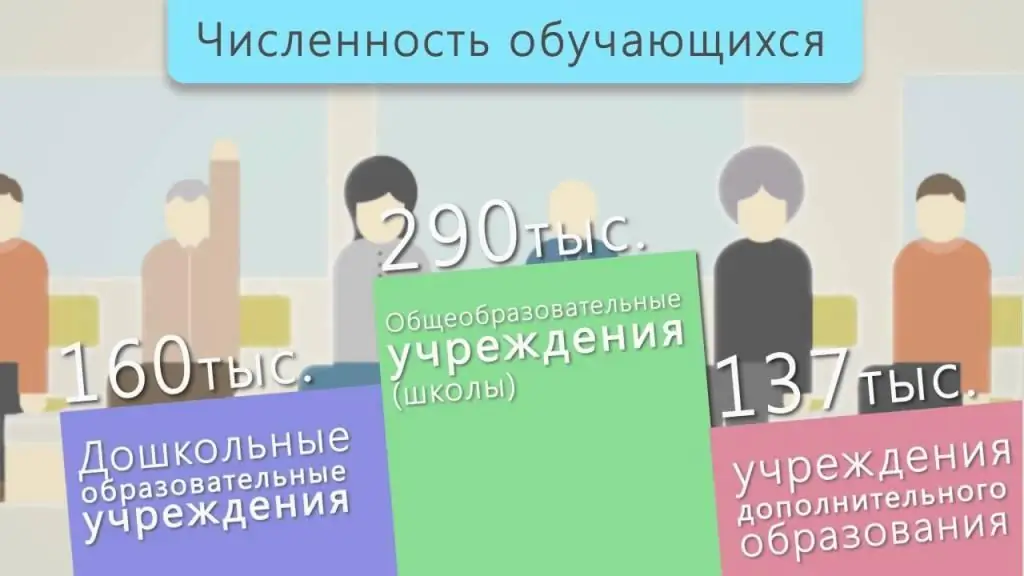
Variables and classification of information
Because the variablescorresponding only to nominal or ordinal measurements cannot reasonably be measured numerically, and are sometimes grouped as categorical variables. The ratio and interval measurements are grouped as quantitative variables, which can be either discrete or continuous due to their numerical nature. Such differences are often loosely related to data type in computer science, since dichotomous categorical variables can be represented by boolean values, polytomous categorical variables with arbitrary integers in an integral data type, and continuous variables with real components that involve floating point computation. But the display of statistical information data types depends on which classification is applied.
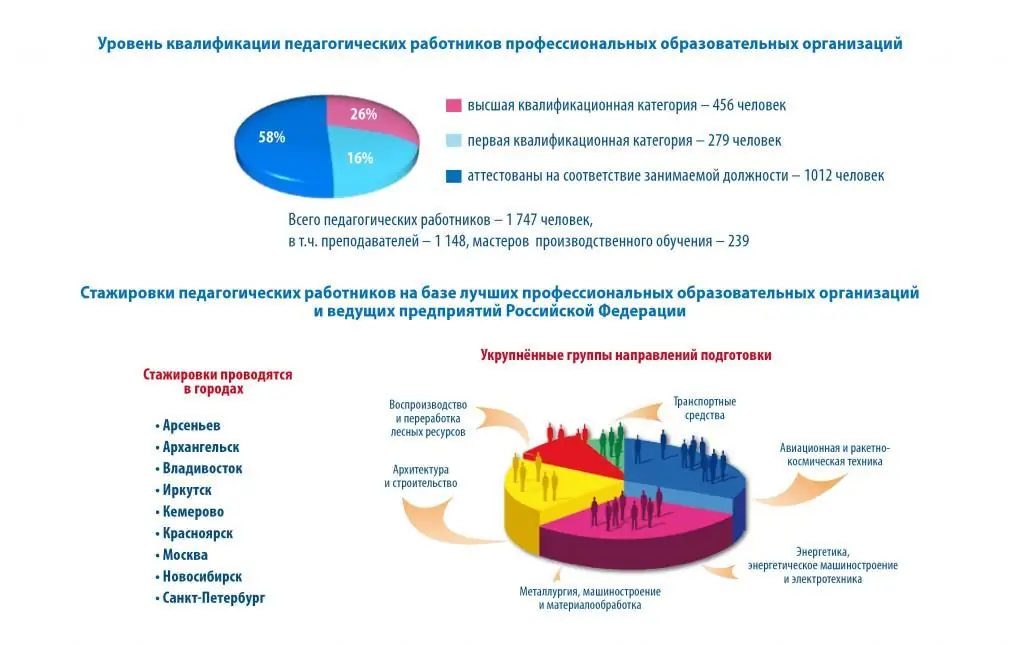
Other classifications
Other classifications of statistical data (information) have also been created. For example, Mosteller and Tukey distinguished between grades, ranks, counted shares, counts, amounts, and balances. Nelder at one time described continuous counts, continuous ratios, correlation of counts, and categorical ways of communicating data. All these classification methods are used in the collection of statistical information.
Problems
The question of whether it is appropriate to apply different kinds of statistical methods to data obtained through different measurement (collection) procedures is complicated by issues relating to the conversion of variables and the precise interpretation of questionsresearch. “The relationship between data and what it describes simply reflects the fact that certain kinds of statistical statements can have truth values that are not invariant under certain transformations. Whether the transformation is worth considering depends on the question you are trying to answer.
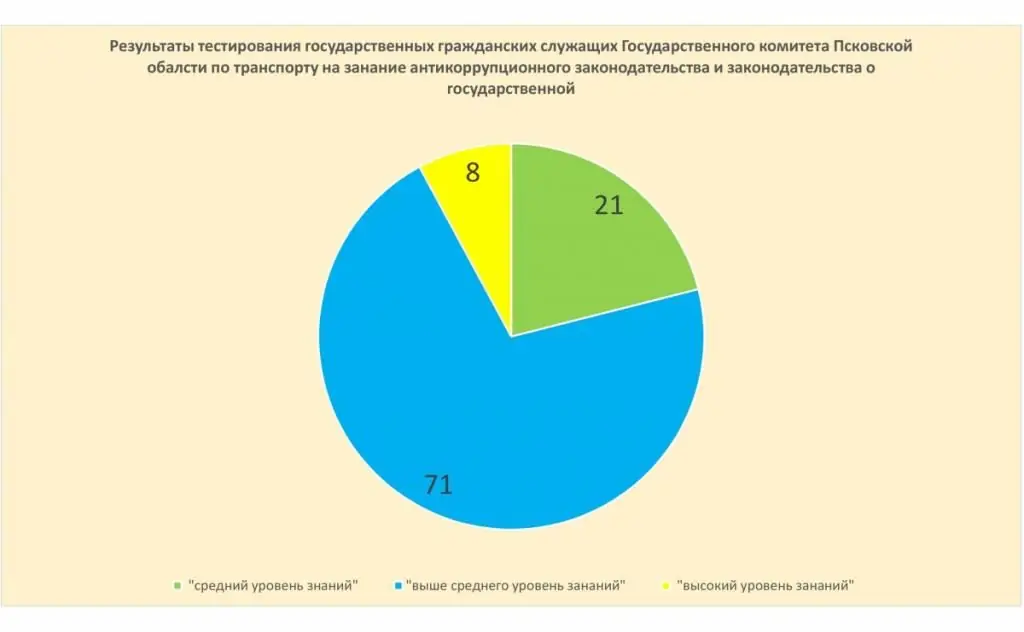
What is a data type
The data type is a fundamental component of the semantic content of a variable and controls what kinds of probability distributions can be logically used to describe the variable, the operations allowed on it, the type of regression analysis used to predict it, etc. The concept of a data type is similar on the concept of measurement level, but more specific - for example, data counts require a different distribution (Poisson or binomial) than for non-negative real values, but both fall under the same level of measurement (coefficient scale).
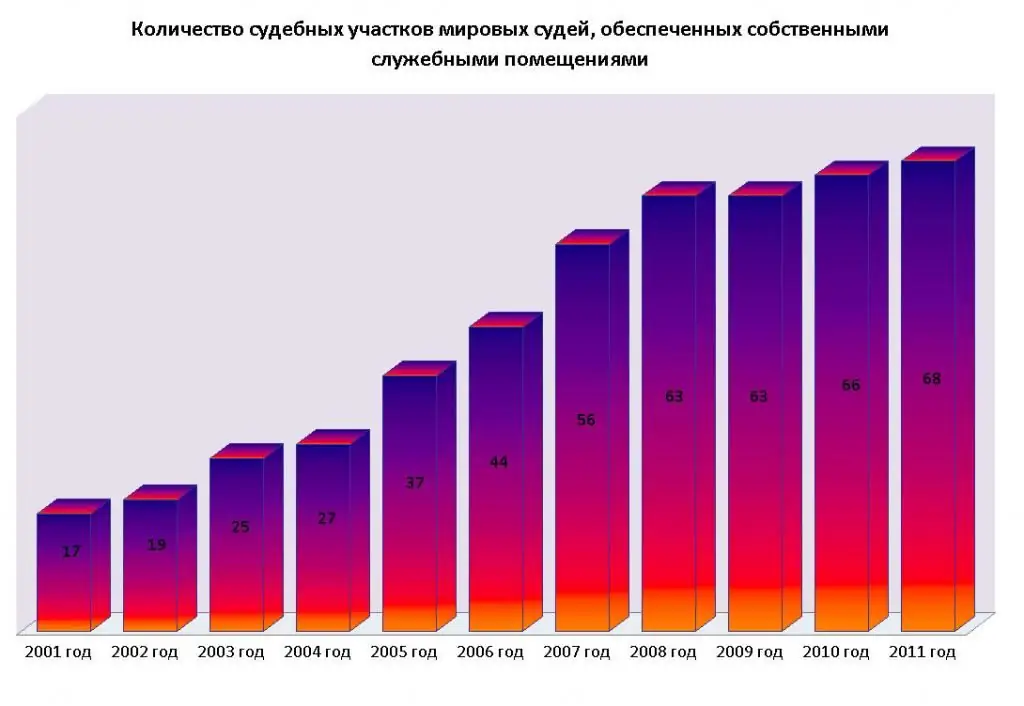
Scales
Various attempts have been made to create a taxonomy of measurement levels for processing statistical information. Psychophysicist Stanley Smith Stevens defined nominal, ordinal, interval, and proportional scales. Nominal measurements do not have a significant order of ranks among the values and allow any one-to-one conversion. Ordinary measurements have imprecise differences between successive values, but differ in the significant order of those values, and allowany order-preserving transformation. Interval measurements have meaningful distances between measurements, but the zero value is arbitrary (as in the case of longitude and temperature measurements in Celsius or Fahrenheit) and allows for any linear transformation. Ratio dimensions have both a meaningful zero value and distances between different defined dimensions, and allow for any scaling transformation.
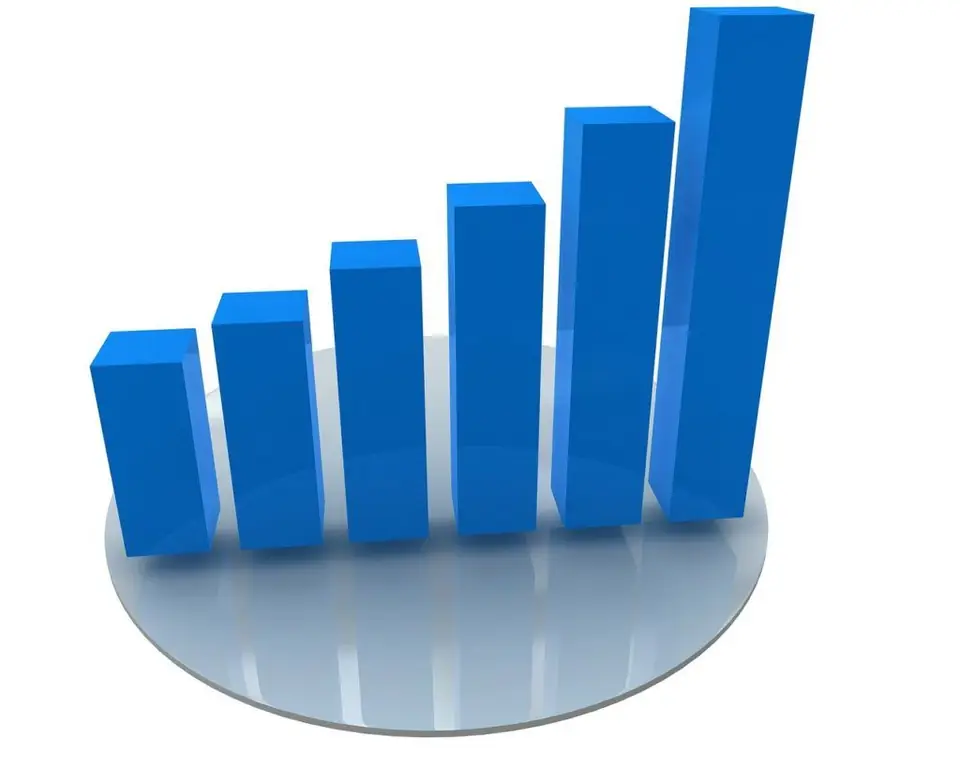
Data that cannot be described using a single number is often included in random vectors of real random variables, although there is a growing trend to process them yourself. Such examples will be discussed below.
Random vectors
Individual elements may or may not be correlated. Examples of distributions used to describe correlated random vectors are the multivariate normal distribution and the multivariate t-distribution. In general, there can be arbitrary correlations between any elements, however this often becomes unmanageable above a certain size, requiring additional constraints on the correlated components.
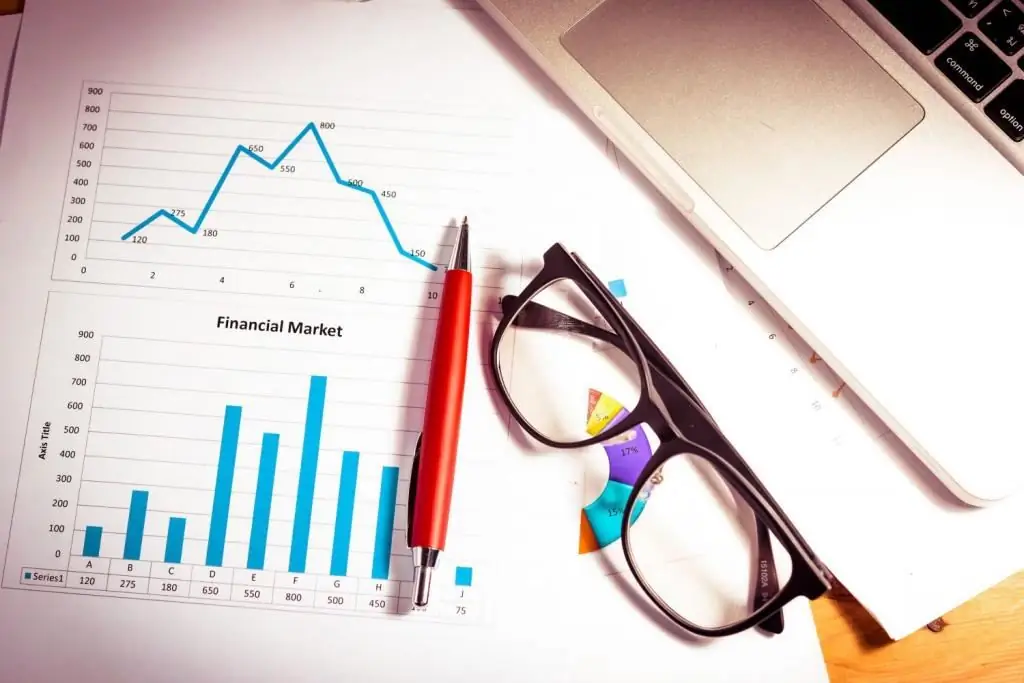
Random matrices
Random matrices can be arranged linearly and treated as random vectors, however this may not be an efficient way to represent correlations between different elements. Some probability distributions are specifically designed for random matrices, such as the normal matrixdistribution and Wishart distribution.
Random Sequences
Sometimes they are considered the same as random vectors, but in other cases the term is applied specifically to cases where each random variable correlates only with nearby variables (as in a Markov model). This is a special case of the Bayesian network and is used for very long sequences, such as gene chains or long text documents. A number of models are specially designed for such sequences, such as hidden Markov sequences.
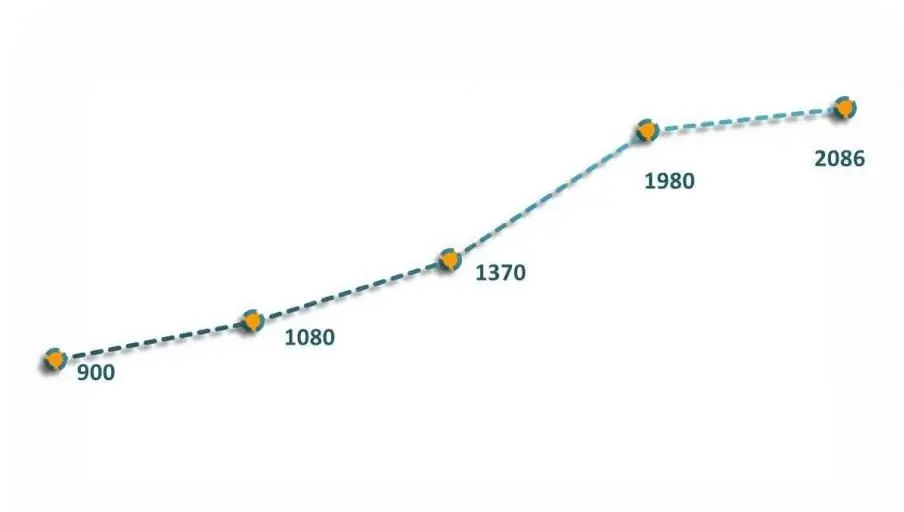
Random processes
They are similar to random sequences, but only when the length of the sequence is indefinite or infinite, and the elements in the sequence are processed one by one. This is often used for data that can be described as time series. This is true when it comes to, for example, the stock price the next day.
Conclusion
The analysis of statistical information entirely depends on the quality of its collection. The latter, in turn, is strongly related to the possibilities of its classification. Of course, there are many types of classification of statistical information, which the reader could see for himself when reading this article. Nevertheless, the presence of effective tools and a good command of mathematics, as well as knowledge in the field of sociology, will do their job, allowing you to conduct any survey or study without significant corrections for error. Sources of statistical information in the formpeople, organizations and other subjects of sociology, fortunately, are represented in great abundance. And no difficulty can stand in the way of a true explorer.